Introduction
Tokenization is a common task in Natural Language Processing (NLP). It’s a fundamental step in both traditional NLP methods like Count Vectorizer and Advanced Deep Learning-based architectures like Transformers.
Table of contents
Source: YouTube | Tokenization.
Tokenization
Tokenization is a way of separating a piece of text into smaller units called tokens. Here, tokens can be either words, characters, or subwords. Hence, tokenization can be broadly classified into 3 types – word, character, and subword (n-gram characters) tokenization.
Also Read: Using artificial intelligence to make publishing profitable.
For example, consider the sentence: “Never lose hope”.
The most common way of forming tokens is based on space. Assuming space as a delimiter, the tokenization of the sentence results in 3 tokens – Never-lose-hope. As each token is a word, it becomes an example of word tokenization.
Similarly, tokens can be either characters or subwords. For example, let us consider “smarter”:
Character tokens: s-m-a-r-t-e-r
Subword tokens: smart-er
As tokens are the building blocks of Natural Language, the most common way of processing the raw text happens at the token level.
Also Read: Democracy will win with improved artificial intelligence.
Transformer
For example, Transformer based models – the State of The Art (SOTA) Deep Learning architectures in NLP – process the raw text at the token level. Similarly, the most popular deep learning architectures for NLP like RNN, GRU, and LSTM also process the raw text at the token level.
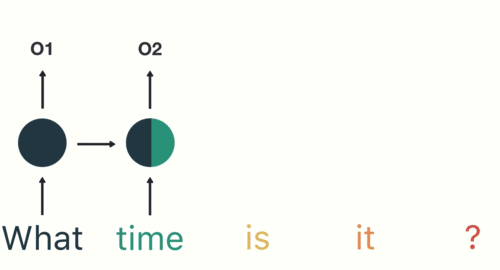
Hence, Tokenization is the foremost step while modeling text data. Tokenization is performed on the corpus to obtain tokens. The following tokens are then used to prepare a vocabulary. Vocabulary refers to the set of unique tokens in the corpus. Remember that vocabulary can be constructed by considering each unique token in the corpus or by considering the top K frequently occurring words.
Also Read: Robotics and manufacturing.
Now, let’s understand the usage of the vocabulary in Traditional and Advanced Deep Learning-based NLP methods.
Traditional NLP approaches such as Count Vectorizer and TF-IDF use vocabulary as features. Each word in the vocabulary is treated as a unique feature:
In Advanced Deep Learning-based NLP architectures, vocabulary is used to create the tokenized input sentences. Finally, the tokens of these sentences are passed as inputs to the model.
Conclusion
Tokenization is a fundamental step in Natural Language Processing (NLP) that influences the performance of high-level tasks such as sentiment analysis, language translation, and topic extraction. It is the process of breaking down text into smaller units, or tokens, such as words or phrases. Tokenization not only simplifies the subsequent processes in the NLP pipeline but also enables the model to understand the context and semantic relationships between words.
Despite its apparent simplicity, tokenization can handle complex linguistic nuances and cater to different languages and text structures. Its importance in NLP can’t be overstated as the quality of tokenization directly impacts the effectiveness of the overall NLP system. As advancements in AI and machine learning continue, more sophisticated tokenization techniques are expected to emerge, enhancing the performance of NLP systems further.
References
Bandler, Richard, et al. The Ultimate Introduction to NLP: How to Build a Successful Life. HarperCollins UK, 2013.