Introduction
Artificial Intelligence and Otolaryngology have come a long way in the past few years. As an ENT surgeon, I decided to pen a few lines on this subject.
Accurate and timely diagnosis of disease continues to be a serious clinical problem in healthcare industry. This is particularly important for Otolaryngology/Ear-Nose-Throat (ENT) disease as ENT disorders can affect hearing, speaking, learning and many other important activities. Further, certain untreated ENT diseases can be fatal. Therefore, early diagnose of ENT diseases is vital for patient care.
While ENT specialist’s service is not always readily accessible, AI aided smart technologies that can assist general physicians or junior medical officers in diagnosing ENT diseases and subsequently refer complicated cases to senior ENT experts can enhance the efficacy of healthcare system.
Table of contents
Also Read: The Impact of Artificial Intelligence in Ophthalmology
Artificial intelligence and otolaryngology, a deep dive.
In the world of audiology and ENT, we have seen the introduction of AI in the area of hearing aid design and feature functionality – striving to provide end users with a transparent and seamless listening experience by predicting and mimicking the decision process of the end user for a given listening condition.
Many global regions are experiencing barriers when it comes to accessing good ENT and audiological services because of the shortage of audiologists and ENT specialists. By virtue of its impact on communication, literacy, and employability, hearing loss is a major risk factor for poverty. Conversely, people living in poverty are more likely to have a hearing loss. Otitis media is the second most common cause of hearing loss and is the most common childhood illness, especially in low- and middle-income countries.
Over 90% of the world’s population experience limited or no access to audiology and ENT services.
Otolaryngology and Telehealth
Artificial intelligence and Otolaryngology can bridge this gap by Telehealth services that can reach a lot of folks who cannot afford quality patient care. Telehealth allows one hearing care professional to service larger populations remotely and in an asynchronous manner. Community-based health workers gather audiological data through screening equipment built for that purpose, and an audiologist can look over the results and recommend a treatment plan for each patient.
It was found that a group of seven healthcare workers, with minimal training, could test 100 children each hour.
The use of AI in accurately classifying otitis media and other conditions has been reported. Image processing could automatically classify otoscopy images at an accuracy of 78% for images captured on-site with a low-cost video otoscope, compared to 80% accuracy achieved for images taken with commercial video otoscopes.
This smartphone- and cloud-based automated otitis media diagnosis system was further improved with the addition of a neural network used for classification of the ear canal and tympanic membrane features.
This improved system demonstrated 87% accuracy in diagnosis. The system allows the user to load a video otoscope-captured tympanic membrane image in a mobile application, and pre-process the image on the smartphone, after which the image is sent to a server where feature extraction and classification/diagnosis is performed. The diagnosis is displayed on the phone of the user.
The HearX group launched the HearScope system with an AI add-on in a beta version.The system offers a low-cost smartphone-based video otoscope that is used to capture images and video of the ear canal and tympanic membrane.
Also Read: Impact Of Automation In Healthcare
The AI function gives an image classification within seconds.of the image for the most common ear conditions.
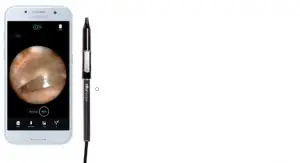
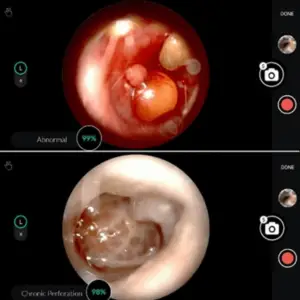
The HearScope system employs two AI systems in classifying otoscopy images. The first screens whether the image is of the ear canal and tympanic membrane. If an ear canal is confirmed, the second neural network classifies the images in one of four categories: normal, wax obstruction, chronic perforations and abnormal.
Based on a large database of images diagnosed with the same category by at least two ENT specialists, the images are classified and abnormal category identified. The abnormal category consists of a number of other specific conditions, such as acute otitis media, that require treatment and can therefore triage patients accordingly.
Also Read: How Can RPA Help In Healthcare?
The average diagnostic accuracy of pediatricians is around 80%, that of GPs is 64–75%, and that of ENTs 73%. The decision tree classification performance was 81.58%, while it was 86.84% when the neural network was used.
The current system that has been released has a 94% accuracy for classifying images into the four currently supported diagnostic categories.
To characterize the tympanic membrane and ear canal for each condition, three different feature extraction methods are used: color coherence vector, discrete cosine transform, and filter bank.
Also Read: How Hospitals Use Algorithms to Prioritize COVID-19 Vaccine Distribution
There are three ML algorithms used for this.
- Support vector machine (SVM),
- K-nearest neighbor (k-NN)
- Decision trees to develop the ear condition predictor model.
The learning models are repeatedly trained using the training dataset and evaluated them using the validation dataset to thus obtain the best feature extraction method and learning model that produce the highest validation accuracy.
Finally a classification stage was performed –i.e., diagnosis using testing data, where the SVM model achieved an average classification accuracy of 93.9%, average sensitivity of 87.8%, average specificity of 95.9%, and average positive predictive value of 87.7% this system might be used by general practitioners.
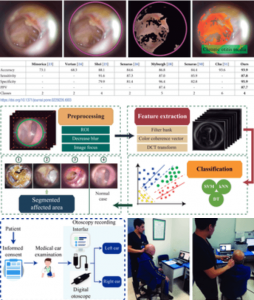
Medical imaging techniques such as CT, MRI, PET scan, ultrasound and X-ray images, have facilitated the creation of large digital databases that can be processed with AI tools. AI is playing an important role in medical imaging interpretation to support tasks such as early detection, accurate diagnosis and treatment for diseases. AI enhances medical tasks and skills, thus overcoming fatigue, distraction, access to new techniques.
Also Read: The Role of Artificial Intelligence in Healthcare Documentation
The most relevant applications of AI involve machine learning (ML) techniques which include enhancing cancer diagnosis especially in metastatic breast cancer detection. improve early detection of polyps to prevent colorectal cancer. with accuracy achieved greater than 98%; and classification of tissues and subsequent recognition of cardiovascular organs.
There are still open challenges when we come to Artificial Intelligence and Otolaryngology. In this context, some efforts are focused on head and neck oncology and the use of ML to classify malignant tissue based on radiographic and histopathologic features. Other studies have been developed for inner ear disorders. Classification of hearing loss phenotypes and vocal fold diagnoses. However, the use of AI tools for external or middle ear diseases is still at early stages of research.
Also Read: Image Annotations: For AI driven Skin Conditions Diagnosis
Some studies have developed computer-aided systems based on a binary classification approach. They used color information of the eardrum image to train different learning models –e.g., decision trees, SVM, neural networks and Bayesian decision approaches– and to predict if the image corresponds to a normal ear or otitis media case.
Furthermore, the classification stage was made using classical SVM approach. A more complete study to classify middle ear diseases was presented in which included specific information for diseases such as tympanosclerosis, perforation, cerumen, retraction and post-injection crust. Other studies proposed smartphone-based diagnostic system.
Also Read: Artificial Intelligence in Healthcare Business Process Improvement
The images were acquired via a portable digital otoscope, then they were stored and visualized on a smartphone as well, but the images were previously processed on a dedicated server. Another related study proposed a deep learning model for detection and segmentation of the structures of the tympanic membrane. Although the aim of such study is not conducted to support or help diagnose, the positive rate detection of structures of the tympanic membrane was the highest reported up to date: 93.1%.
Recently, convolutional neural networks (CNNs) were used diagnose otitis media. it employed a transfer learning approach to classify between four classes: normal, acute otitis media, chronic suppurative otitis media, and wax.
The two main drawbacks needs sufficient data to generate a reproducible model and model bias due to the image acquisition process –only one device was used to acquire the images. Later a CNN model to binary classification was developed between normal and otitis media cases, and also to analyze the presence or absence of perforation on the tympanic membrane achieving an accuracy of 91%.
Also Watch: Healed through A.I. | The Age of A.I. | S1 | E2
Ear imagery database
Ear examinations were carried out using a digital otoscope DE500 Firefly –with a 0.4 mm and 0.5 mm speculums– connected to a computer. We acquired the video files at 20 FPS (frames per second) with a 640×480 pixels resolution.
The physician was capable of visualizing the video acquired by the digital otoscope in real-time, on the computer screen. a user interface was developed for the specialist, to minimize operational delays associated with the use of the equipment to record the physician’s diagnosis.
Also Read: The role of AI in vaccine distribution
Data pre-processing
Based on the physician’s diagnosis, 90 video otoscopies are selected for each condition –wax, myringoesclerosis and chronic otitis media– and the same number of videos for normal cases. Considering the images being in RGB format, images of the eardrum the frames of each recording were analyzed to extract best frames. After selecting the best frames from each video, ROI that included the eardrum were isolated.
Features extraction
In this a texture descriptor, is created i.e., the frequency histogram as features to train a machine learning models are used. To compute the frequency histogram, created a texton dictionary is created. For this purpose, multiple images from a set of a specific class were convolved with the filter bank.
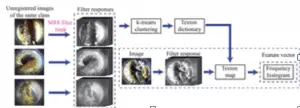
Also Read: Artificial Intelligence in Healthcare.
Machine learning models for artificial intelligence and otolaryngology.
- Support vector machine.
- K-nearest neighbors.
- Decision trees.
Such techniques are considered as supervised learning algorithms that learn from a training set of examples with the associated correct responses often provide a high classification performance on reasonably sized datasets. To generate a prediction model, two sets of data were used: the training set and the validation set.
Support vector machine (SVM).
A support vector machine is one of the most popular algorithms in modern machine learning Training an SVM model consists of searching the largest radius around a classification boundary (margin) where no data points are placed. The closets points to this margin are called support vectors and are used to define a decision boundary in the classification problem.
K-nearest neighbors (k-NN).
The k-nearest neighbor is often selected in classification problems where there is no prior knowledge about the distribution of the data. To make a prediction for a test example, it first computes the distance between such test example and each point in the training set. Then, the algorithm keeps the closest training examples (k number of examples) and looks for the label that is most common among these examples.
Decision trees (DT).
In decision trees approach classification is carried out by a set of choices about each feature in turn, starting at the root (base) of the tree and processing down to the leaves, where the algorithm delivers the classification decision.
Evaluation metrics
Performance of classification models can be measured from computing a confusion matrix. The confusion matrix indicates the number of instances which corresponds to the predicted and the actual classes. This concept is often used in binary classification, but it can be extended to a multi-class prediction where the corresponding class is located on the diagonal of the matrix.
To evaluate the learning models, computed accuracy that can be obtained from confusion matrix scores is saved.other evaluations metrics such as sensitivity, specificity and positive predictive value are considered. The definitions of these metrics in a binary classification approach are extended to multi-class classification using macro-averaging method.
- Positive predictive value—PPV (often called precision) is the ratio between the correctly classified instances from a given class and the total number of instances classified as belonging to that class.
- Receiver operating characteristic—ROC is typically used in binary classification to evaluate the performance of a classifier. It also has been widely used to compare the performance between several classifiers Results
To obtain a system capable of assisting the physician’s diagnosis, a pair of: feature extraction method with learning model that produces the highest validation accuracy, sensitivity, specificity are selected.
A learning model is generated using 720 images (180 per class). To perform the classification tests, randomly split the images into a training set.and a validation set
Such random splitting was performed 100 times,For each split, the machine learning models are trained using data set and evaluated each model performance on the validation data set. and the evaluation metrics are summarized for –i.e., accuracy, sensitivity, specificity and positive predictive value.
It was observed that both the SVM and k-NN outperform the decision trees model in terms of accuracy, sensitivity, specificity and PPV. The highest validation accuracy (99.0%) is achieved when the system uses the filter-bank as a feature extraction method and SVM as the learning model.
The k-NN model achieved a close but lower performance than SVM. The decision trees achieved a lower performance with validation accuracy of 93.5%, sensitivity of 86.9%, specificity of 95.6% and PPV of 87.3%.
The proposed system is capable to highlight the area if the result of the prediction model was any of three ear conditions: earwax plug., myringosclerosis and chronic otitis media.
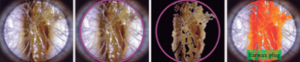
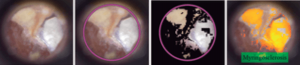
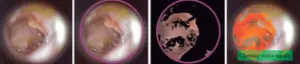
- Image 1. From left to right: RGB input image, region of interest, wax segmentation and area under medical suspicion highlighted.
- Image 2. Myringosclerosis the system highlights the white areas found in the tympanic membrane.
- Image 3. Chronic otitis media case: the system highlights the perforated area denoted by red color.
Also Read: How can Artificial Intelligence help with the Coronavirus (Covid-19) vaccine search?
Conclusion and future work
ENT diseases affect the daily lives of the patients, both adults and children suffer. Some of the diseases have serious impact in the developing countries; it can cause a challenged language development and progress among the children. There are the most common childhood infection leading to annual death of over 50,000 children under 5 years.
Artificial intelligence and otolaryngology can help save lives and improve overall patient care. AI will be a great assistive technology for doctors, especially ENT specialists to cater to a spectrum of medical challenges in ENT care, which at this point is not accessible to many. AI’s help in enabling the ENT specialists to treat patients remotely with robotics will be a game changer in the healthcare.
References
“hearScope by hearX Group – Digital Video Otoscope with AI Image Classification.” hearX Group, https://www.hearxgroup.com/hearscope/. Accessed 4 June 2023.
Kim, Stefani. “HearX to Release Beta Version of HearScope AI Classification Feature.” The Hearing Review, 9 Mar. 2020, https://www.hearingreview.com/hearing-products/testing-equipment/testing-diagnostics-equipment/hearx-to-release-beta-version-of-hearscope-ai-classification-feature. Accessed 4 June 2023.
Pedersen, Jenny Elise Nesgaard. “Digital Otoscopy with AI Diagnostic Support: Making Diagnosis of Ear Disease More Accessible.” ENT & Audiology News, 2 June 2020, https://www.entandaudiologynews.com/development/spotlight-on-innovation/post/digital-otoscopy-with-ai-diagnostic-support-making-diagnosis-of-ear-disease-more-accessible. Accessed 4 June 2023.